- Developed a computer vision algorithm for analyzing user-uploaded test strip photos.
- Goal was to create a reliable algorithm with accurate results and a user-friendly experience.
- Built a simple windows desktop app for OraStripDX to test the algorithm.
Computer Vision Test Strip Analysis For Pet Health
Training a computer vision algorithm to quickly provide accurate, real-time feedback.
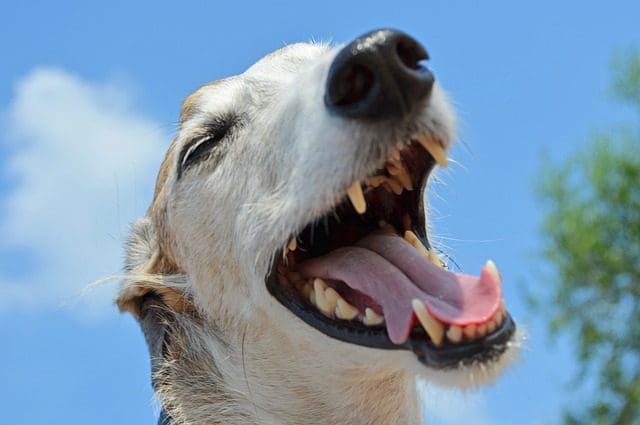
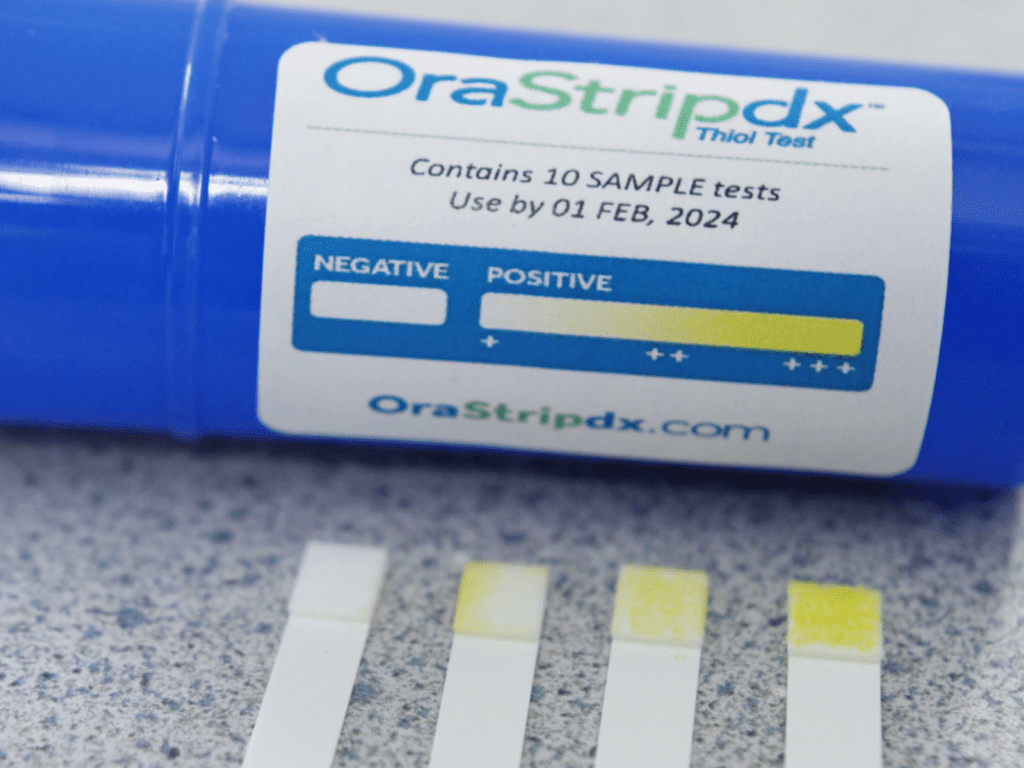
The Story
OraStripDX has developed a 10-second test to detect periodontal disease in pets. The test is conducted by rubbing the strip against your pet's teeth, and waiting for the results. This disease is a major cause of early tooth loss in animals. They came to Slingshot wanting to create a computer vision algorithm that could simplify the process of evaluating test results for users. To achieve this, they utilized computer vision and AI technologies to analyze photos of test strips. The ultimate goal was to create a user-friendly solution enabling non-technical and non-medical users to interpret test results accurately, thus expanding their customer base.
Technology: Computer Vision, AI, AWS services
The Story
OraStripDX has developed a 10-second test to detect periodontal disease in pets. The test is conducted by rubbing the strip against the dogs tooth, and waiting for the results. This disease is a major cause of early tooth loss in animals. They came to Slingshot wanting to create a computer vision algorithm that could simplify the process of evaluating test results for users. To achieve this, they utilized computer vision and AI technologies to analyze photos of test strips. The ultimate goal was to create a user-friendly solution enabling non-technical and non-medical users to interpret test results accurately, thus expanding their customer base.
Technology: Computer Vision, AI, AWS services
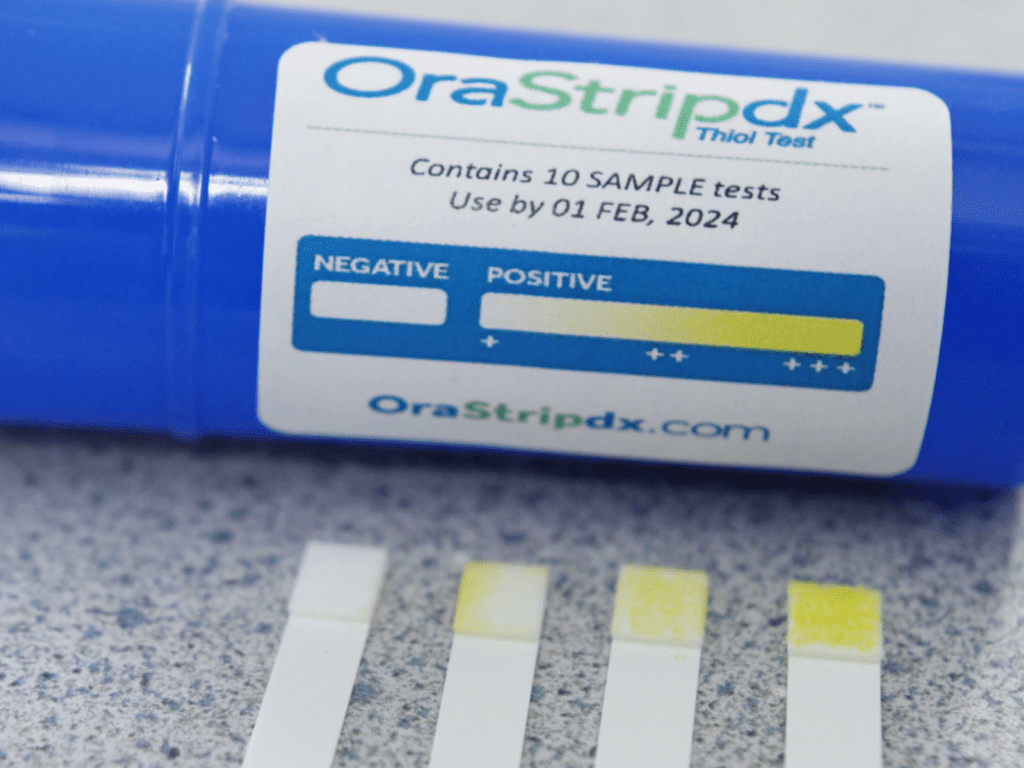
Challenges
After we began testing the images, we realized we would have to account for lighting issues. Since the test strips turn yellow with a positive result, we needed to balance the scan if the user was in a yellow-tinged or warm-light room. The solution was to have the user include the bottle's result tracker in the photo as a reference point. That way, we could balance the colors and get an accurate result.
Key Features
Hitting The Target
The project successfully concluded with the deployment of the computer vision algorithm integrated into the simple windows desktop app. Users can upload a photo of a test strip and receive real-time answers interpreted by the algorithm. We eliminated the need for users to manually match colors or analyze the results themselves. The project's outcome paves the way for a mobile app that can make the user experience even easier!
~99%
Accuracy of algorithm